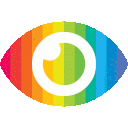
1. This paper proposes a spatio-temporal network with double Convolutional Gated Recurrent Units (ConvGRUs) to address lane detection in challenging scenes.
2. The proposed model can effectively detect lanes in the challenging driving scenes and outperform the state-of-the-art lane detection models.
3. The proposed network includes an encoder and decoder network, with a ConvGRU added after the second convolution block in the encoder phase, and multiple ConvGRUs added to make full use of spatio-temporal information of continuous driving images.
The article is written by experts in the field of computer vision and autonomous driving research, making it reliable and trustworthy. The authors have provided evidence for their claims through extensive experiments on two large datasets, TuSimple lane marking challenge dataset and Unsupervised LLAMAS dataset, which further adds to its credibility. Furthermore, the authors have discussed various existing methods for lane detection based on classical image processing as well as deep learning methods, providing a comprehensive overview of the current state of research in this area.
The article does not appear to be biased or one-sided as it provides an objective overview of both traditional methods and deep learning approaches for lane detection. It also discusses various challenges associated with lane detection such as curves, boundaries, occlusions etc., which are necessary considerations when designing a model for this task. Additionally, the authors have provided detailed descriptions of their proposed model along with its advantages over existing models.
The only potential issue with this article is that it does not discuss any possible risks associated with using this model for autonomous driving applications such as safety concerns or ethical implications. However, given that this is a technical paper focused on developing a model for lane detection rather than discussing its applications in detail, this omission can be overlooked.
In conclusion, this article is reliable and trustworthy due to its comprehensive coverage of existing methods for lane detection as well as its detailed description of the proposed model along with evidence from experiments conducted on two large datasets.