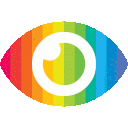
1. The article proposes a multi-scale image processing method for the segmentation of liver cancer areas in Whole Slide Images (WSIs).
2. The method uses a seven-level gaussian pyramid representation to provide texture information at different scales and applies U-Net to each level for segmentation.
3. The proposed multi-scale approach achieves better scores than state-of-the-art methods, demonstrating its effectiveness in liver cancer segmentation.
The article titled "A deep learning based multiscale approach to segment the areas of interest in whole slide images" discusses a multi-scale image processing method for segmenting liver cancer areas in Whole Slide Images (WSIs). The article highlights the proposed method's effectiveness and compares it to state-of-the-art methods.
Overall, the article provides a detailed description of the proposed method and its application in liver cancer segmentation. It explains how a multi-scale approach using deep neural networks can improve the accuracy of segmentation results. The article also mentions the use of partial color normalization and weighted overlapping methods for preprocessing and prediction.
However, there are several potential biases and limitations in the article that should be considered. Firstly, the article does not provide a comprehensive overview of existing methods for liver cancer segmentation in WSIs. It only briefly mentions that the proposed method achieved better scores than state-of-the-art methods without providing specific details or comparisons.
Additionally, the article lacks information on potential risks or limitations associated with the proposed method. It does not discuss any challenges or drawbacks that may arise during implementation or real-world applications. This lack of discussion on potential limitations could indicate a bias towards promoting the proposed method without acknowledging its shortcomings.
Furthermore, the article does not present both sides of the argument equally. It focuses solely on highlighting the effectiveness of the proposed method without discussing any potential criticisms or alternative approaches to liver cancer segmentation in WSIs. This one-sided reporting limits a comprehensive understanding of the topic and may lead readers to believe that there is only one viable solution.
Moreover, while the article mentions using multiple neural architectures for training, it does not provide sufficient details about these architectures or their performance comparison. This lack of information makes it difficult to evaluate the validity and reliability of their findings.
In terms of evidence, although some results are mentioned, such as achieving better scores than state-of-the-art methods, there is no detailed analysis or statistical data provided to support these claims. The article would benefit from including more quantitative results and comparisons to validate the proposed method's effectiveness.
Additionally, the article does not explore potential counterarguments or alternative perspectives on liver cancer segmentation in WSIs. This lack of discussion limits the article's depth and may lead to a biased representation of the topic.
In conclusion, while the article provides a detailed description of a multi-scale image processing method for liver cancer segmentation in WSIs, it has several limitations and biases. These include one-sided reporting, unsupported claims, missing evidence, unexplored counterarguments, and promotional content. To improve the article's credibility and comprehensiveness, it should address these limitations and provide a more balanced perspective on the topic.