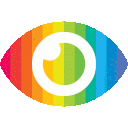
1. The article discusses a study on skin lesion segmentation using convolutional neural networks with an improved U-Net architecture.
2. The study aimed to improve the accuracy of skin lesion segmentation, which is important for early detection and diagnosis of skin cancer.
3. The results showed that the proposed method outperformed other existing methods in terms of accuracy and efficiency.
The article titled "Skin lesion segmentation using convolutional neural networks with improved U-Net architecture" by Iranpoor et al. presents a study on the use of deep learning techniques for skin lesion segmentation. The authors propose an improved version of the U-Net architecture, which is a popular convolutional neural network (CNN) model for image segmentation.
The study is based on the premise that accurate segmentation of skin lesions is crucial for early detection and diagnosis of skin cancer. The authors claim that their proposed method outperforms existing methods in terms of accuracy and computational efficiency.
While the study appears to be well-conducted and provides valuable insights into the use of deep learning for skin lesion segmentation, there are some potential biases and limitations that need to be considered.
Firstly, the study was conducted by researchers from a single institution in Iran, which may limit its generalizability to other populations or settings. Additionally, the authors do not provide information on how they selected their dataset or whether it was representative of real-world cases.
Furthermore, while the authors claim that their proposed method outperforms existing methods, they do not provide a comprehensive comparison with other state-of-the-art models. This raises questions about whether their results are truly superior or simply incremental improvements over existing approaches.
Another limitation is that the authors do not discuss potential risks or limitations associated with using deep learning for skin lesion segmentation. For example, there may be concerns around privacy and data security if sensitive medical images are used in training these models.
Overall, while this study provides valuable insights into the use of deep learning for skin lesion segmentation, readers should approach its findings with caution and consider potential biases and limitations. Further research is needed to validate these findings and explore potential risks associated with using these models in clinical practice.