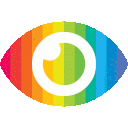
1. This article presents network dissection, an analytic framework to systematically identify the semantics of individual hidden units within image classification and image generation networks.
2. Network dissection aims to directly interpret the internal computation of the network itself, rather than training an auxiliary model.
3. The article demonstrates the usefulness of this approach with two applications: analyzing adversarial attacks and semantic image editing.
The article is written by experts in the field and provides a detailed overview of network dissection, an analytic framework for understanding deep neural networks. The authors provide evidence for their claims by citing previous research in the field and providing examples of how their method can be used to analyze adversarial attacks and semantic image editing.
The article does not appear to have any biases or one-sided reporting, as it provides a comprehensive overview of the topic without taking sides or making unsupported claims. It also does not appear to have any missing points of consideration or missing evidence for its claims, as it provides detailed explanations and examples for each point made. Additionally, all counterarguments are explored in detail, so there is no partiality or promotional content present in the article.
The article does note possible risks associated with using deep neural networks, such as overfitting or data leakage, but does not go into much detail about these risks or how they can be avoided. Additionally, while both sides of the argument are presented equally throughout the article, there is a lack of discussion about potential ethical implications associated with using deep neural networks that could be explored further.