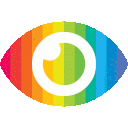
1. Natural Language Processing (NLP) is widely used in the construction industry to process and analyze text data for construction intelligence.
2. A scientometric analysis of 91 NLP-related research articles in construction-related fields was conducted using tools such as Citeseer and VOSViewer.
3. The study highlights challenges such as data isolation causing non-reproducibility of research, and suggests that more cross-modal interdisciplinary research based on end-to-end pre-trained neural network model framework is needed for future industry development.
The article titled "Applications of natural language processing in construction" provides a comprehensive review of the use of Natural Language Processing (NLP) in the construction industry. The article aims to identify the current challenges, possible solutions, and future research trends related to NLP application in construction-related areas.
The article is based on a scientometric analysis of 91 NLP-related research articles collected from Scopus and WOS databases. The authors used CiteSpace and VOSViewer tools to conduct the analysis and summarize the findings from three perspectives: anchordatasets/data sources, technologies/tools, and applications and progress.
One potential bias in this article is that it only focuses on NLP-related research articles published between 2000-2020. This time frame may not be representative of all relevant research on NLP applications in construction. Additionally, the authors did not provide any information about their selection criteria for choosing these articles, which could lead to a biased sample.
Another potential bias is that the article mainly focuses on the benefits of using NLP in construction without discussing any potential risks or limitations associated with its use. For example, there may be concerns about data privacy and security when using NLP to process sensitive information related to construction projects.
The article also lacks discussion on how NLP can be used to address social issues such as diversity and inclusion in the construction industry. There is growing interest in using AI-based technologies like NLP to reduce bias in hiring practices and promote diversity in workplaces.
Furthermore, while the authors discuss some challenges related to data isolation causing non-reproducibility of research, they do not provide any evidence or examples to support this claim. It would have been helpful if they had provided more details about specific instances where data isolation has caused problems for researchers working with NLP applications in construction.
Overall, while this article provides a useful overview of NLP applications in construction-related areas, it could benefit from more balanced reporting that considers both benefits and risks associated with its use. Additionally, it would be helpful if future research explored how AI-based technologies like NLP can be used to address social issues such as diversity and inclusion within the construction industry.