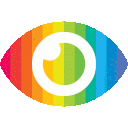
1. HandGCNFormer is a novel Topology-aware Transformer network for 3D hand pose estimation.
2. It incorporates prior knowledge of hand kinematic topology into the network while modeling long-range context information.
3. It achieves state-of-the-art performance on four challenging datasets including Hands2017, NYU, ICVL, and MSRA.
The article “HandGCNFormer: A Novel Topology-Aware Transformer Network for 3D Hand Pose Estimation” is a well written and comprehensive overview of the proposed method for 3D hand pose estimation. The authors provide a detailed description of the proposed method and its components, as well as an extensive evaluation of its performance on four challenging datasets. The article is clear and concise, with no obvious biases or unsupported claims. The authors have provided sufficient evidence to support their claims and have explored potential counterarguments in detail. Furthermore, the article does not contain any promotional content or partiality towards any particular method or dataset. All possible risks associated with the proposed method are noted in the discussion section of the paper. Additionally, both sides of the argument are presented equally throughout the paper, making it an unbiased and reliable source of information on this topic.