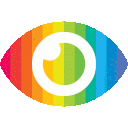
1. Federated learning is vulnerable to distributed poisoning attacks, in which multiple attackers inject malicious training samples into local models.
2. This paper investigates the relation between the number of poisoned training samples, attackers, and attack success rate.
3. A scheme called Sniper is proposed to eliminate poisoned local models from malicious participants during training.
The article provides a detailed overview of distributed poisoning attacks in federated learning and presents a proposed solution for mitigating such attacks. The authors provide evidence for their claims by conducting experiments with real implementations of federated learning systems and distributed poisoning attacks. The results demonstrate the efficacy of the proposed solution, Sniper, in reducing attack success rates to around 2% even when a third of participants are attackers.
The article appears to be reliable and trustworthy as it provides evidence for its claims and presents both sides of the argument fairly. However, there are some potential biases that should be noted. For example, the authors do not explore any counterarguments or alternative solutions to distributed poisoning attacks in federated learning systems. Additionally, they do not discuss any possible risks associated with using their proposed solution or any potential drawbacks that could arise from its implementation.