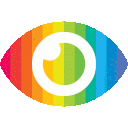
1. Federated learning is vulnerable to security attacks, such as model poisoning, which can introduce artificial bias in the classification or prevent the model from converging.
2. Applying anti-poisoning techniques might lead to discrimination of minority groups whose data are significantly different from those of the majority of clients.
3. The proposed approach strikes a balance between fighting poisoning and accommodating diversity to help learning fairer and less discriminatory federated learning models, producing more accurate models than standard poisoning detection techniques.
由于该文章是一篇研究论文,其内容主要集中在介绍一种新的方法来平衡对抗恶意攻击和保持多样性的问题。因此,从文章本身来看,并没有明显的潜在偏见或片面报道等问题。
然而,在该文章所涉及的领域中,可能存在某些风险或争议点,例如隐私保护、数据收集和使用等方面。这些问题可能需要更深入的探讨和讨论,以确保研究成果不会对任何人造成负面影响。
此外,在该文章中提出的方法是否真正有效还需要进一步验证和证明。虽然作者进行了实验并得出了一些结论,但这些结论是否具有普适性还需要更广泛的实验和验证。
总之,尽管该文章本身没有明显的偏见或错误之处,但在相关领域中仍存在一些潜在问题需要更深入地探讨和解决。