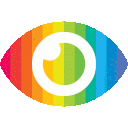
1. The face unlocking feature on smartphones using regular 2D camera sensors performs poorly in low light environments.
2. A semi-supervised low light face enhancement method has been proposed to improve face verification performance on low light face images.
3. The proposed method uses a network with two components: decomposition and reconstruction, and is trained using both labeled synthetic data and unlabeled real data. It reduces the gap of verification accuracy between extreme low light and neutral light face images from approximately 3% to 0.5%.
The article titled "SeLENet: A Semi-Supervised Low Light Face Enhancement Method for Mobile Face Unlock" discusses a proposed method to improve face verification performance on low light face images. The article highlights the importance of facial recognition as a standard feature on new smartphones and the poor performance of face unlocking in low light environments using regular 2D camera sensors.
The proposed method is a network with two components: decomposition and reconstruction. The decomposition component splits an input low light face image into face normals and face albedo, while the reconstruction component enhances and reconstructs the lighting condition of the input image using the spherical harmonic lighting coefficients of a direct ambient white light. The network is trained in a semi-supervised manner using both labeled synthetic data and unlabeled real data.
The article provides qualitative results demonstrating that the proposed method produces more realistic images than state-of-the-art low light enhancement algorithms. Quantitative experiments confirm the effectiveness of the low light face enhancement method for face verification, reducing the gap of verification accuracy between extreme low light and neutral light face images from approximately 3% to 0.5%.
While the article presents a promising solution to improve face verification performance in low light environments, it may have some biases and limitations. Firstly, it focuses solely on improving smartphone technology rather than considering potential risks associated with facial recognition technology, such as privacy concerns or bias against certain demographics. Additionally, it does not explore counterarguments or alternative solutions to improving facial recognition in low light environments.
Furthermore, while the article claims that its proposed method outperforms state-of-the-art algorithms, it does not provide sufficient evidence or comparisons with other methods to support this claim fully. Additionally, there may be potential biases in how data was collected or labeled for training purposes.
Overall, while the proposed method shows promise in improving facial recognition technology in low light environments, further research is necessary to address potential biases and limitations and consider broader implications beyond technological advancements.