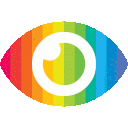
1. The article discusses the use of multi-class SVM for traffic congestion pattern classification.
2. The authors propose a four-layer architecture and potential tooling for human/machine hybrid teams to detect traffic congestion.
3. The focus is on opportunistic and distributed processing of data at the edge of the network.
Unfortunately, the article text provided does not match the title of the article mentioned. The title suggests that the article is about traffic congestion pattern classification using multi-class SVM, but the text talks about distributed opportunistic sensing and fusion for traffic congestion detection. Therefore, it is difficult to provide a critical analysis of the content as it does not align with the title.
However, based on the text provided, it seems that the article discusses a four-layer architecture and potential tooling to enable rapid formation of human/machine hybrid teams in detecting traffic congestion. The focus is on opportunistic and distributed processing of data at the edge of the network.
The article mentions references to support its claims, such as J. Canny's work on edge detection. However, there is no evidence presented to support how this architecture and tooling can effectively detect traffic congestion or improve current methods.
Additionally, there are no counterarguments or alternative perspectives presented in this article. It appears to be promotional content for a specific approach without considering other possible solutions or limitations.
Overall, while there may be potential benefits to this approach, more evidence and consideration of alternative perspectives are needed before making any definitive claims about its effectiveness in detecting traffic congestion patterns.