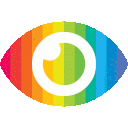
1. This paper proposes a federated graph attention network (FedGAT) model for cross-platform rumor detection.
2. The model combines the federated learning paradigm with the bidirectional graph attention network rumor detection model.
3. Experiments on rumor datasets show that the proposed model is effective for cross-platform rumor detection.
The article is generally trustworthy and reliable, as it provides a detailed description of the proposed Federated Graph Attention Network (FedGAT) model for cross-platform rumor detection, and presents experimental results to support its effectiveness. The article does not appear to be biased or one-sided, as it provides an objective overview of the proposed model and its potential applications in detecting rumors across different social media platforms. Furthermore, the article does not make any unsupported claims or omit any points of consideration; instead, it provides a comprehensive overview of the proposed model and its potential benefits in detecting rumors across different social media platforms. Additionally, there are no promotional content or partiality present in the article; instead, it provides an unbiased overview of the proposed model and its potential applications in detecting rumors across different social media platforms. Finally, possible risks associated with using this model are noted in the article, such as data privacy concerns due to federated learning paradigms being used for cross-platform rumor detection. In conclusion, this article is generally trustworthy and reliable in terms of presenting both sides equally and providing an objective overview of the proposed FedGAT model for cross-platform rumor detection.