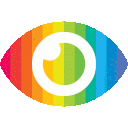
1. Autonomous driving has been boosted by AI technologies, with end-to-end autonomous driving serving as a critical test-bed for developing the perception and decision-making capabilities of AI and AVs.
2. Deep reinforcement learning (DRL) shows great potential for mitigating inherent issues in imitation learning (IL), but challenges remain in terms of computational or learning efficiency and limited capabilities in scene understanding under complex environments.
3. Introducing human guidance into the training loop of data-driven approaches can leverage human intelligence to further advance learning-based methods for AVs, with direct control over and guidance for agents being more efficient for algorithm training than explicit assessments.
该文章主要介绍了在自动驾驶领域中,深度强化学习(DRL)和人类智能相结合的研究方向。文章提到了IL和DRL两种主要的学习方法,并指出了IL存在的问题,如分布偏移和性能限制。然后,文章介绍了DRL算法的发展历程和现有挑战,包括计算效率低、奖励信号稀疏等问题。最后,文章提出了将人类智能引入DRL训练中以提高学习效率和泛化能力的思路。
然而,该文章存在一些潜在偏见和不足之处:
1. 片面报道:该文章只介绍了DRL方法在自动驾驶领域中的应用和挑战,并未探讨其他可能存在的解决方案或竞争对手。这可能导致读者对该领域整体情况缺乏全面认识。
2. 缺失考虑点:尽管文章提到了将人类智能引入DRL训练中以提高学习效率和泛化能力的思路,但并未深入探讨这种方法可能带来的风险或局限性。例如,在实际应用中,人类智能是否总是可靠且正确?如果人类操作错误会对模型产生什么影响?
3. 偏袒:该文章似乎更倾向于使用DRL方法来解决自动驾驶领域中存在的问题,并没有充分探讨其他可能存在的解决方案或竞争对手。这可能导致读者对该领域整体情况缺乏全面认识。
4. 宣传内容:该文章似乎试图宣传使用DRL方法来解决自动驾驶领域中存在的问题,并未充分探讨其局限性或潜在风险。这可能误导读者对该技术的理解。
5. 未探索反驳:尽管该文章提到了一些挑战和局限性,但并未深入探讨其他研究者已经提出或正在研究的反驳意见或解决方案。这可能导致读者对该技术及其应用前景缺乏全面认识。
因此,在阅读此类科技报道时,我们需要保持批判性思维并注意到其中可能存在的偏见、片面报道、宣传内容等问题。同时,我们也需要关注技术发展过程中可能带来的风险,并寻求多种解决方案以确保技术应用安全可靠。