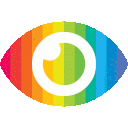
1. The use of machine learning for automatic surface anomaly detection has become a promising research field with high direct impact on visual inspection applications.
2. A segmentation-based deep learning architecture was proposed for detecting and segmenting surface anomalies, specifically in the domain of surface crack detection.
3. The proposed method outperformed related methods in the specific domain of surface crack detection, and was able to learn from a small number of defective training samples, making it applicable to industries with limited defective sample availability.
The article discusses a deep learning method for surface defect detection based on segmentation. The authors claim that their proposed method outperforms other deep learning methods and commercial software in detecting surface cracks. However, the article lacks some important details and evidence to support these claims.
One potential bias in the article is that it only focuses on the strengths of the proposed method and does not mention any limitations or weaknesses. For example, the authors do not discuss how their method performs on surfaces with different textures or colors, or how it handles complex shapes or patterns. Additionally, they do not provide any information about false positive rates or how their method compares to human inspection.
Another issue with the article is that it does not provide enough information about the dataset used for training and testing. The authors state that they created a new dataset for real-world quality control cases, but they do not describe how they collected or labeled this data. Without this information, it is difficult to assess the validity and generalizability of their results.
The article also contains some unsupported claims, such as when the authors state that deep learning methods are "the most suitable" for surface defect detection without providing any evidence to support this assertion. Additionally, while they claim that their method requires fewer training samples than other approaches, they do not explain why this is the case or provide any evidence to back up this claim.
Overall, while the proposed deep learning method may have potential for surface defect detection, more research and evidence are needed to fully evaluate its effectiveness and limitations. The article could benefit from a more balanced discussion of both its strengths and weaknesses, as well as more detailed information about the dataset used for testing and validation.