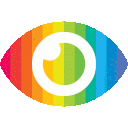
1. The concept of "urban vitality" refers to the intensity of people's concentration in a city and is considered the goal of good urban design.
2. Mobile phone data has become an ideal data source for characterizing human activity patterns and measuring urban vitality from a continuous perspective of time and space quantitatively.
3. A clustering method based on a neuron network model was designed to mine the spatiotemporal features of urban vitality, providing a reliable basis for urban infrastructure construction and optimal allocation of resources.
The article "Urban Vitality Area Identification and Pattern Analysis from the Perspective of Time and Space Fusion" provides a comprehensive analysis of the concept of urban vitality and its measurement using big data. The article highlights the importance of understanding the spatiotemporal dynamic characteristics of urban human spatial activities and their relationships with urban resource allocation to provide a reliable basis for urban infrastructure construction and optimal allocation of resources.
The article presents a clustering method based on a neuron network model to mine the spatiotemporal features of urban vitality. The method is applied to identify and analyze vitality areas in Nanjing, China, using mobile phone data as a data source. The analysis results provide a realistic reference value for evaluating the current situation of urban construction and future urban planning.
While the article provides valuable insights into measuring urban vitality using big data, it has some potential biases. For instance, it focuses only on one city, Nanjing, which may not be representative of other cities globally. Additionally, while mobile phone data is an ideal data source for characterizing human activity patterns, it may not capture all aspects of human activity accurately.
Moreover, the article does not explore counterarguments or limitations to its findings. For example, while the clustering method used in this study may be effective in identifying vitality areas in Nanjing, it may not be applicable to other cities with different characteristics.
Furthermore, the article does not discuss possible risks associated with collecting and analyzing big data or potential ethical concerns related to privacy violations. It also lacks information on how bias was controlled during data collection and analysis.
In conclusion, while the article provides valuable insights into measuring urban vitality using big data, it has some potential biases that need to be considered when interpreting its findings. Further research is needed to validate these findings across different cities globally and address potential ethical concerns related to privacy violations.