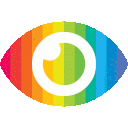
1. Chemoproteomic probes coupled with mass spectrometry can be used to globally profile protein features and interrogate the mode of action of small molecules in a native biological system.
2. Blind search informatic tools, in combination with isotope-coding approaches for probes, can provide an unbiased survey of probe-derived modifications that can be distinguished from unmodified or endogenously modified ones.
3. pChem is an automated, user-friendly computational tool designed to provide a pipeline for rapid and unbiased assessment of the performance of activity-based protein profiling and metabolic labeling probes. It generates all possible modifications using bona fide blind search on the basis of a tag-index approach and produces modification-centric, constructive, and easy-to-interpret outputs for probe developers.
The article titled "A modification-centric assessment tool for the performance of chemoproteomic probes" published in Nature Chemical Biology discusses the development of a computational tool called pChem that can be used to assess the performance of chemoproteomic probes. The article highlights the challenges associated with developing an efficient and selective probe for chemoproteomics, including unbiasedly assessing its chemoselectivity at a proteome-wide scale and unforeseeable probe-derived modifications (PDMs) that may yield during sample preparation or in-source MS fragmentation.
The article provides a detailed description of how pChem works, starting experimentally by isotopic coding of PDMs, which can be automatically recognized, paired, and accurately reported by pChem. The tool then allows users to score the profiling efficiency, modification homogeneity, and proteome-wide residue selectivity of chemoproteomic probes. The article also discusses the challenges associated with blind search tools and how pChem addresses these challenges.
Overall, the article is well-written and provides valuable insights into the development of pChem as a computational tool for assessing the performance of chemoproteomic probes. However, there are some potential biases and limitations to consider.
One potential bias is that the article focuses primarily on the benefits of using pChem without discussing any potential risks or limitations associated with its use. For example, it is unclear whether there are any potential drawbacks or limitations to using pChem in certain experimental settings or whether there are any concerns about false positives or false negatives when using this tool.
Another limitation is that while the article provides a detailed description of how pChem works, it does not provide any evidence to support its claims about the effectiveness or accuracy of this tool. For example, there is no discussion about how well pChem performs compared to other existing tools for assessing chemoproteomic probes or whether there are any known limitations or weaknesses in its approach.
Additionally, while the article briefly mentions some previous studies that have used similar blind search tools for assessing chemoproteomic probes, it does not provide a comprehensive overview of this field or discuss any potential counterarguments or alternative approaches.
In conclusion, while this article provides valuable insights into the development of pChem as a computational tool for assessing chemoproteomic probes, it is important to consider its potential biases and limitations. Further research will be needed to fully evaluate the effectiveness and accuracy of this tool in different experimental settings and to address any concerns about false positives or false negatives.