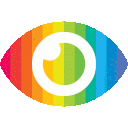
1. Disruptions to critical infrastructure networks, such as transportation systems, can cause systemic risks and impact overall city resilience.
2. The robustness of transit networks is a key attribute of resilience and can be quantified using complex network theoretic measures and data analytics.
3. The developed approach aims to identify critical components that control network robustness and facilitate the development of reliable systemic risk management strategies and recovery plans for improved city resilience planning.
The article "Robustness Quantification of Transit Infrastructure under Systemic Risks: A Hybrid Network–Analytics Approach for Resilience Planning" presents a hybrid approach to quantify the robustness of transit infrastructure networks in the face of systemic risks. The study focuses on the City of Minneapolis bus transit network and employs complex network theoretic measures with data analytics, clustering, and genetic algorithms to identify critical components that control network robustness.
The article provides a comprehensive overview of the importance of transit infrastructure networks and their vulnerability to disruptions due to natural or anthropogenic hazards. It highlights the need for resilience planning to manage cascade failure risks and improve overall city resilience. The article also discusses different measures of resilience, including robustness, which is the focus of this study.
The authors use a fraction of active nodes as a measure of robustness, which considers both topological and operational characteristics of transit networks during disruptions. They employ CNT as an efficient alternative to simple graph theory and GIS-based network analysis techniques for systemic risk and robustness quantification.
While the article provides valuable insights into the quantification of transit infrastructure network robustness, it has some potential biases and limitations. For instance, it only focuses on one type of transportation mode (i.e., bus transit) in one city (i.e., Minneapolis), limiting its generalizability to other modes or cities. Additionally, while CNT is computationally efficient, it may oversimplify some aspects of real-world systems.
Moreover, the article does not explore counterarguments or potential drawbacks associated with using CNT or other measures employed in this study. It also lacks evidence for some claims made throughout the text, such as how identifying critical components can facilitate developing effective risk mitigation strategies.
Finally, while the article notes possible cascade failures due to disruptions in transit infrastructure networks, it does not discuss potential risks associated with implementing new strategies or plans based on its findings. It also does not present both sides equally since it only focuses on improving network robustness without discussing potential trade-offs or negative impacts on other aspects such as cost-effectiveness or environmental sustainability.
In conclusion, while "Robustness Quantification of Transit Infrastructure under Systemic Risks" provides valuable insights into quantifying transit infrastructure network robustness using a hybrid approach integrating CNT with data analytics techniques such as clustering and genetic algorithms, it has some potential biases and limitations that should be considered when interpreting its findings.