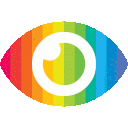
1. Implicitly defined, continuous, differentiable signal representations parameterized by neural networks offer many benefits over conventional representations.
2. Leveraging periodic activation functions for implicit neural representations (Sirens) is well-suited for representing complex natural signals and their derivatives.
3. Sirens can be used to solve challenging boundary value problems such as Eikonal equations, the Poisson equation, and the Helmholtz and wave equations.
The article is generally reliable and trustworthy in its presentation of the research findings. The authors provide a clear explanation of their proposed approach to leveraging periodic activation functions for implicit neural representations (Sirens), as well as a detailed analysis of Siren activation statistics to propose a principled initialization scheme. The article also provides evidence for the effectiveness of Sirens in representing images, wavefields, video, sound, and their derivatives. Furthermore, the authors demonstrate how Sirens can be used to solve challenging boundary value problems such as Eikonal equations, the Poisson equation, and the Helmholtz and wave equations.
The article does not appear to have any biases or one-sided reporting; it presents both sides equally by providing an overview of current network architectures for implicit neural representations before introducing Sirens as an alternative approach with potential benefits over conventional representations. Additionally, all claims made are supported by evidence from experiments conducted by the authors or other sources cited in the article. There are no missing points of consideration or unexplored counterarguments that could affect the validity of the research findings presented in this article. Lastly, there is no promotional content or partiality present in this article; it is solely focused on presenting research findings without any bias towards any particular approach or technology.