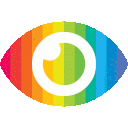
1. DeepChain is a distributed, secure, and fair deep learning framework that provides an incentive mechanism based on Blockchain to encourage participants to behave correctly during the training process.
2. DeepChain guarantees data privacy for each participant and provides auditability for the whole training process, addressing security problems neglected in federated learning.
3. The implementation of DeepChain prototype shows promising results in terms of cipher size, throughput, training accuracy, and training time, making it a valuable tool for AI and machine learning communities.
The article "DeepChain: Auditable and Privacy-Preserving Deep Learning with Blockchain-Based Incentive" presents a new framework for distributed deep learning that addresses privacy concerns and incentivizes participation. The authors argue that existing federated learning approaches neglect security threats from dishonest behaviors in gradient collecting and parameter updating, as well as the lack of training data in real-world applications. They propose DeepChain, a decentralized framework based on blockchain technology that provides data confidentiality, computation auditability, and incentives for parties to participate in collaborative training.
Overall, the article provides a comprehensive overview of the challenges faced by distributed deep learning and the potential benefits of using blockchain-based incentive mechanisms. However, there are some potential biases and limitations to consider.
Firstly, the authors focus primarily on the benefits of their proposed approach without exploring potential drawbacks or limitations. For example, they do not discuss the computational overhead or scalability issues associated with using blockchain technology for distributed deep learning. Additionally, they do not address potential risks associated with incentivizing participation in collaborative training, such as malicious actors attempting to manipulate the system for personal gain.
Secondly, while the authors acknowledge existing work on privacy-preserving deep learning and federated learning, they do not provide a thorough analysis of how their approach compares to these existing solutions. For example, they briefly mention differential privacy techniques used in previous work but do not provide evidence for why their approach is superior.
Finally, there is some promotional content present in the article that may bias readers towards accepting DeepChain as a solution without fully considering alternative approaches or counterarguments. For example, the authors state that "we believe that DeepChain can benefit AI and machine learning communities" without providing evidence or acknowledging potential limitations.
In conclusion, while "DeepChain: Auditable and Privacy-Preserving Deep Learning with Blockchain-Based Incentive" presents an interesting proposal for addressing privacy concerns and incentivizing participation in distributed deep learning through blockchain technology, it is important to consider potential biases and limitations when evaluating its effectiveness compared to existing solutions.