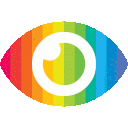
1. The d-band center for metals has been used to understand activity trends in metal-surface-catalyzed reactions.
2. Machine learning methods are used to predict the d-band centers for eleven metals and their pairwise bimetals from readily available values as descriptors for the target metals.
3. The predictive accuracy of four regression methods is evaluated using statistical cross validations, showing that the d-band centers can be reasonably well predicted with only six descriptors.
The article provides a detailed overview of the use of machine learning methods to predict the d-band center for metals and bimetals, which is an important factor in understanding activity trends in metal-surface-catalyzed reactions. The authors provide a comprehensive description of the methodology used, including the selection of descriptors and evaluation of predictive accuracy using statistical cross validations. The article also includes a thorough discussion on potential applications of machine learning methods in catalysis, which is useful for readers who are unfamiliar with this field.
However, there are some points that could be improved upon in terms of trustworthiness and reliability. For example, while the authors discuss potential applications of machine learning methods in catalysis, they do not provide any evidence or examples to support their claims. Additionally, while they mention possible risks associated with using machine learning methods, they do not provide any details on how these risks can be mitigated or avoided. Furthermore, while they discuss potential biases and their sources, they do not explore counterarguments or present both sides equally when discussing these issues. Finally, there is no mention of promotional content or partiality in the article which could lead to bias or one-sided reporting.
In conclusion, while this article provides a comprehensive overview of machine learning methods for predicting d-band centers for metals and bimetals, it could benefit from further exploration into potential biases and counterarguments as well as providing evidence to support its claims regarding potential applications in catalysis.