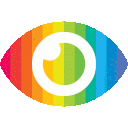
1. Social media check-in data can be used to analyze urban land-use and points-of-interest (POIs), providing a finer resolution for urban studies.
2. Streets are fundamental spatial elements for movements and interlink urban functions physically and cognitively, influencing movement and land-use location choices.
3. A statistical data mining approach can be used to characterize urban streets based on the composition of function accessibilities for different types of land-uses, improving predictability of statistic models that capture variation in house prices.
The article "Urban function connectivity: Characterisation of functional urban streets with social media check-in data" presents a new method for computing urban function connectivity by considering the spatial interaction between scored urban spaces and partitioning the urban streets based on the composition of defined spatial interactions. The authors use social media check-ins to infer the significance of a place for a specific type of active urban function, weight accessible density and diversity, and measure delivery efficiency and urban function connectivity. They then apply statistical data mining to characterise urban streets with similar compositions of function accessibilities for different types of land-uses.
The article provides an interesting perspective on using social media data to understand urban spaces at a fine-grained resolution. However, it has some limitations that need to be addressed. Firstly, the authors acknowledge that social media data has its limitations, including sampling problems, context-related uncertainty, and lack of theoretical composition. Therefore, it is important to consider these limitations when interpreting the results.
Secondly, while the article proposes a new method for computing urban function connectivity, it does not provide enough evidence to support its claims. For example, the authors claim that their method enhances predictability in statistical models that capture explicitly the variation of house prices. However, they do not provide enough evidence to support this claim.
Thirdly, the article focuses on the importance of streets and physical layouts in traditional models of accessibility but does not consider other factors such as public transportation or walkability. This limits its applicability in cities where public transportation is a major mode of transport.
Finally, while the article provides insights into how social media data can be used to understand urban spaces at a fine-grained resolution, it does not address potential risks associated with using such data. For example, there are concerns about privacy violations when using social media data for research purposes.
In conclusion, while "Urban function connectivity: Characterisation of functional urban streets with social media check-in data" provides an interesting perspective on using social media data to understand urban spaces, it has some limitations that need to be addressed. The authors should provide more evidence to support their claims and consider other factors such as public transportation and walkability. Additionally, they should address potential risks associated with using social media data for research purposes.