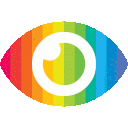
1. PixelNeRF is a neural radiance field model that can generate 3D scenes from one or few images.
2. The official repository for the paper includes environment setup instructions, data download links, and commands for running the model on different datasets.
3. The provided evaluation code allows for both approximate and full evaluation of the model's performance on various datasets.
The article discusses the official repository for the paper "pixelNeRF: Neural Radiance Fields from One or Few Images" by Alex Yu, Vickie Ye, Matthew Tancik, and Angjoo Kanazawa from UC Berkeley. The article provides information on how to set up the environment, obtain data, and run the model for various experiments.
Overall, the article appears to be informative and well-organized. However, there are some potential biases and missing points of consideration that should be noted. Firstly, the article does not provide any information on potential limitations or drawbacks of the pixelNeRF model. While it is understandable that this may not be the focus of the article, it would have been helpful to include some discussion on potential risks or limitations associated with using this model.
Additionally, there is a lack of discussion on potential biases in the data used for training and testing the model. For example, it is unclear whether there are any biases in the ShapeNet dataset used for experiments. This could potentially impact the generalizability of results obtained from using this model.
Furthermore, while the article provides detailed instructions on how to run various experiments using pixelNeRF, there is no discussion on potential counterarguments or alternative approaches to achieving similar results. This could potentially lead readers to believe that pixelNeRF is the only viable approach for these types of experiments.
Finally, it should be noted that this article appears to be promotional in nature as it is promoting a specific research paper and its associated repository. While this is not necessarily a negative aspect of the article itself, readers should keep in mind that there may be other approaches or models available that are not discussed in this particular article.
In conclusion, while this article provides useful information on running experiments using pixelNeRF and its associated repository, readers should keep in mind potential biases and limitations associated with using this model and dataset. Additionally, readers should consider alternative approaches and potential counterarguments when evaluating the usefulness of pixelNeRF for their own research purposes.