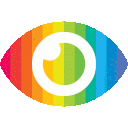
1. Sarcasm detection in social media is important for sentiment analysis and identifying cyberbullies.
2. Deep learning-based approaches have achieved high performance but lack interpretability.
3. The proposed approach uses multi-head self-attention to enable interpretability while achieving state-of-the-art results on various datasets.
The article "Interpretable Multi-Head Self-Attention Architecture for Sarcasm Detection in Social Media" presents a deep learning-based approach to detect sarcasm in social media texts. The authors argue that the presence of sarcasm hinders sentiment analysis, and therefore, it is important to develop models that can identify sarcastic cues in the input text. They propose a model that uses multi-head self-attention and gated recurrent units to learn long-range dependencies between cue words and achieve state-of-the-art results on multiple datasets.
Overall, the article provides a comprehensive overview of the existing literature on sarcasm detection and highlights the limitations of previous approaches. The authors argue that handcrafted features lack generalizability, while deep learning-based methods lack interpretability. Therefore, they propose a model that leverages self-attention to enable interpretability while achieving high performance.
However, there are some potential biases and limitations in the article. Firstly, the authors do not provide a clear definition of what they consider as sarcasm. While they mention that sarcasm is an expression used to communicate the opposite of what is said with an intention to insult or ridicule, they do not provide any examples or criteria for identifying sarcastic expressions. This lack of clarity may lead to subjective interpretations of what constitutes sarcasm.
Secondly, the authors do not discuss the potential risks associated with using automated models for sarcasm detection. For example, automated models may misclassify non-sarcastic expressions as sarcastic or vice versa, leading to incorrect conclusions about public opinion or sentiment towards a particular topic or brand.
Thirdly, while the authors claim that their proposed model achieves state-of-the-art results on multiple datasets, they do not provide any evidence or comparison with other state-of-the-art models. This lack of comparison makes it difficult to assess whether their proposed model is indeed superior to existing approaches.
Finally, while the authors highlight the importance of interpretability in their proposed model, they do not discuss how their model's interpretability compares with other existing interpretable models for sarcasm detection. This omission limits our understanding of how their proposed approach contributes to advancing interpretable machine learning research.
In conclusion, while "Interpretable Multi-Head Self-Attention Architecture for Sarcasm Detection in Social Media" presents an interesting approach to detecting sarcasm in social media texts using deep learning techniques and attention mechanisms, there are some potential biases and limitations in the article that need further consideration.