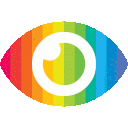
1. Vegetation index-based remote sensing is a standard tool in Earth system science, with NDVI being the most commonly used index.
2. Three types of reflectance are used to calculate NDVI: top-of-atmosphere (TOA), top-of-canopy (TOC), and normalized reflectance with bidirectional reflectance distribution function (BRDF) modeling.
3. The study evaluated the suitability of different NDVI estimation methods using Himawari-8/AHI data, considering atmospheric and BRDF correction, and compared the results with MODIS and PROBA NDVIs.
The article "Evaluation of NDVI Estimation Considering Atmospheric and BRDF Correction through Himawari-8/AHI" discusses the use of vegetation indices in remote sensing and focuses on the normalized difference vegetation index (NDVI). The article highlights the importance of NDVI in various fields, including global change, carbon cycle, land coverage/use, and terrestrial ecology research. The authors compare three types of reflectance to calculate NDVI: top-of-atmosphere (TOA), top-of-canopy (TOC), and normalized reflectance with bidirectional reflectance distribution function (BRDF) modeling.
The article provides a detailed description of the data used in the study, including Himawari-8/Advanced Himawari Imager (AHI) data, cloud mask data for aerosol products provided by the Japan Meteorological Agency (JMA), snow cover data provided by the National Meteorological Satellite Center (NMSC), solar zenith angle (SZA), solar azimuth angle (SAA), viewing zenith angle (VZA), and viewing azimuth angle (VAA) for the angle calculation module acquired from the Communication, Ocean, Meteorological Satellite (COMS)/Meteorological Imager (MI), total ozone column, total precipitable water, and aerosol optical depth provided by the European Centre for Medium-Range Weather Forecasts (ECMWF)/Copernicus Atmosphere Monitoring Service (CAMS).
The authors also describe their method for estimating three types of NDVIs based on TOA reflectance, TOC reflectance, and normalized reflectance. They compared these NDVIs to determine which was most suitable for Himawari-8. They analyzed temporal and spatial differences between the acquired NDVIs by land type and selected an optimal NDVI representation that showed consistent quality without missing values or low peaks.
Overall, this article provides a comprehensive analysis of NDVI estimation considering atmospheric and BRDF correction through Himawari-8/AHI. However, the article does not provide a thorough discussion of potential biases or limitations in the study. The authors do not explore counterarguments or present both sides equally, which could lead to a one-sided reporting of the results. Additionally, the article does not note any possible risks associated with using NDVI in remote sensing or discuss any potential negative impacts on the environment or society.
In conclusion, while this article provides valuable insights into NDVI estimation through Himawari-8/AHI, it would benefit from a more critical analysis of potential biases and limitations in the study. The authors should also consider exploring counterarguments and presenting both sides equally to provide a more balanced perspective on their findings.