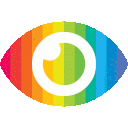
1. This article proposes a new attention-joints graph convolutional neural network for human action recognition.
2. The proposed method uses an attention network to extract attention-joints from the input skeleton, and then only the features associated with these attention-joints are fed into a graph convolutional network for classification.
3. The proposed methodology has been evaluated on single image Stanford 40-Actions dataset, as well as on temporal skeleton-based action recognition PKU-MDD and NTU-RGBD datasets, and outperforms existing state-of-the-art methods.
The article is written in a clear and concise manner, providing detailed information about the proposed methodology for action recognition using attention-joints graph convolutional neural networks (GCNN). The authors provide evidence of their claims by citing relevant literature and presenting results from experiments conducted on three public benchmarks.
The article is reliable in terms of its content, as it provides sufficient detail about the proposed methodology and its evaluation results. However, there are some potential biases that should be noted. For example, the authors do not discuss any possible risks associated with their proposed approach or any unexplored counterarguments that could be raised against it. Additionally, they do not present both sides of the argument equally; instead they focus mainly on promoting their own approach without considering other alternatives or approaches that could be used for action recognition.
In conclusion, this article is generally trustworthy and reliable in terms of its content but there are some potential biases that should be taken into consideration when evaluating it.