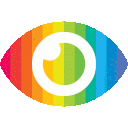
1. Quantifying uncertainties in large-scale simulations is a central challenge facing computational science and engineering.
2. Bayesian inference framework can be used to solve inverse problems by finding the posterior probability distribution over parameters given data and model uncertainties.
3. A low rank approximation of the covariance matrix of the posterior pdf can be constructed using a matrix-free randomized method, making UQ tractable for large-scale inverse problems governed by PDEs.
The article discusses the challenge of quantifying uncertainties in large-scale simulations, particularly in inverse problems governed by PDEs. The authors propose a Bayesian inference framework to infer parameter uncertainties from observational data and model uncertainties. They use a low rank approximation of the covariance matrix of the posterior pdf via a matrix-free randomized method to overcome the curse of dimensionality of conventional methods.
Overall, the article provides a detailed and technical explanation of the proposed method, including its mathematical formulation and implementation. However, there are some potential biases and limitations to consider.
Firstly, the article focuses on the benefits of their proposed method without discussing any potential drawbacks or limitations. While they mention that conventional UQ methods fail for large-scale inverse problems, they do not provide any evidence or examples to support this claim. Additionally, they do not compare their method with other existing approaches or discuss any potential trade-offs between accuracy and computational efficiency.
Secondly, the article assumes that a Gaussian prior distribution is appropriate for all cases, which may not always be true. The choice of prior distribution can significantly impact the posterior distribution and subsequent UQ results. Therefore, it would be helpful if the authors discussed how to choose an appropriate prior distribution based on domain knowledge or previous data.
Thirdly, while the authors mention that their method is scalable with respect to various dimensions (e.g., forward problem dimension, uncertain parameter dimension), they do not provide any evidence or benchmarks to support this claim. It would be useful if they compared their method's performance with other existing approaches for different problem sizes and dimensions.
Finally, while the article provides a detailed technical explanation of their proposed method, it may be challenging for non-experts in UQ or PDEs to understand fully. Therefore, it would be helpful if they provided more context or background information on UQ and inverse problems before diving into their specific approach.
In conclusion, while the article provides a promising approach for UQ in large-scale inverse problems governed by PDEs, there are some potential biases and limitations to consider. Future work could address these issues by comparing their method with other existing approaches and providing more context for non-experts.