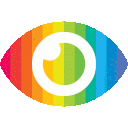
1. Sentiment analysis on Twitter is important for businesses to establish effective campaign ideas and understand public opinion.
2. Three different approaches have been used in sentiment analysis: lexicon-based, standard machine learning-based, and deep learning-based.
3. The proposed model in this study incorporates transfer learning with ULMFiT and SVM to improve the accuracy of classification and reduce training time.
The article provides a comprehensive overview of sentiment analysis on Twitter and the different approaches used to solve this problem. However, there are some potential biases and limitations in the article that need to be addressed.
Firstly, the article focuses mainly on machine learning-based approaches for sentiment analysis and does not provide enough information about other methods such as lexicon-based or unsupervised approaches. This may lead readers to believe that machine learning is the only effective method for sentiment analysis, which is not necessarily true.
Secondly, the article promotes ULMFiT as a universal transfer learning technique for sentiment analysis without discussing its limitations or potential drawbacks. While ULMFiT has been shown to be effective in some cases, it may not always be the best approach for every dataset or task.
Thirdly, the article does not explore counterarguments or alternative perspectives on sentiment analysis. For example, some researchers have argued that sentiment analysis can be biased or inaccurate due to cultural differences or linguistic nuances.
Finally, the article does not address potential risks associated with using sentiment analysis in business or marketing contexts. For example, relying too heavily on automated sentiment analysis could lead companies to overlook important feedback from customers or miss opportunities for improvement.
Overall, while the article provides a useful overview of sentiment analysis on Twitter and machine learning-based approaches, it would benefit from a more balanced discussion of alternative methods and potential limitations and risks associated with this technology.