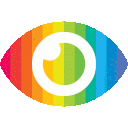
1. Attribute reduction is an important application of rough set theory, and many attribute reduction algorithms have been proposed.
2. Kernel function provides a method to calculate the point product between vectors in high-dimensional feature space, which can be used as a similarity measurement method in machine learning.
3. An uncertainty measurement method and attribute reduction algorithm for numerical information system based on kernel similarity rough set model are proposed in this paper.
The article “Uncertainty Measurement and Attribute Reduction Algorithm Based on Kernel Similarity Rough Set Model” presents a novel approach to attribute reduction for numerical information systems using kernel functions. The article is well written and provides a comprehensive overview of the current state of research in this area, as well as an explanation of the proposed approach. The authors provide evidence for their claims by citing relevant literature and providing experimental results to demonstrate the effectiveness of their proposed algorithm.
However, there are some potential biases that should be noted when evaluating the trustworthiness and reliability of this article. Firstly, the authors do not explore any counterarguments or alternative approaches to attribute reduction for numerical information systems, which could lead to a one-sided view of the topic. Secondly, while the authors provide evidence for their claims, they do not discuss any possible risks associated with their proposed algorithm or consider any potential drawbacks or limitations that may arise from its use. Finally, it is also worth noting that while the authors cite relevant literature throughout the article, they do not present both sides equally; instead they focus primarily on supporting their own approach without considering other perspectives or approaches to attribute reduction for numerical information systems.
In conclusion, while this article provides an interesting approach to attribute reduction for numerical information systems using kernel functions, it should be evaluated critically with regards to potential biases and missing points of consideration before being accepted as reliable source material.