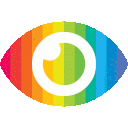
1. Doublets, which are formed when two cells are encapsulated in a single droplet during scRNA-seq experiments, can confound downstream analysis and affect the accuracy of results.
2. Nine computational methods have been developed to detect doublets in scRNA-seq data, but there is a lack of comprehensive benchmark testing to determine their performance and suitability for specific analyses.
3. A systematic benchmark study of these nine methods found that while no method dominates in all aspects, the DoubletFinder method has the best detection accuracy and the cxds method has the highest computational efficiency.
The article titled "Benchmarking Computational Doublet-Detection Methods for Single-Cell RNA Sequencing Data" provides a systematic benchmark study of nine computational doublet-detection methods for scRNA-seq data. The authors evaluate the methods' detection accuracy, impacts on downstream analyses, and computational efficiency using a comprehensive set of real and synthetic data.
The article is well-written and provides valuable insights into the performance of different doublet-detection methods. However, there are some potential biases and limitations that need to be considered.
Firstly, the authors only evaluate nine computational doublet-detection methods, which may not represent all available methods in the field. Therefore, the results may not be generalizable to other methods that were not included in the study.
Secondly, the authors do not provide a detailed explanation of how they selected the 16 real datasets used in their study. It is unclear whether these datasets were randomly selected or if they were biased towards certain cell types or experimental conditions.
Thirdly, while the authors compare different methods' detection accuracy and computational efficiency, they do not consider other important factors such as sensitivity, specificity, and false discovery rate. These factors are crucial when evaluating doublet-detection methods' performance.
Fourthly, the authors do not explore potential counterarguments or limitations of their study's findings. For example, it is possible that some methods perform better on specific cell types or experimental conditions than others.
Finally, while the article does not contain any promotional content or one-sided reporting, it is essential to note that some of the authors have developed one of the evaluated doublet-detection methods (DoubletFinder). This potential conflict of interest should be considered when interpreting their findings.
In conclusion, while this article provides valuable insights into different computational doublet-detection methods' performance for scRNA-seq data analysis, it is essential to consider its potential biases and limitations when interpreting its findings. Future studies should aim to address these limitations by evaluating more comprehensive sets of datasets and considering additional factors such as sensitivity and specificity.