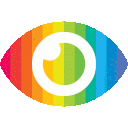
1. Characteristics of medical images: Medical images have relatively simple semantics and fixed structures, making both high-level semantic information and low-level features important. Medical images also often have multiple modalities, requiring better network design to extract feature features of different modalities.
2. U-Net architecture: U-Net is an encoder-decoder structure with skip connections and a U-shaped structure that allows for seamless segmentation of images of any size through an overlapping tiling strategy. It uses data augmentation with elastic deformation to learn the invariance of such deformations without seeing them in a corpus of annotated images.
3. 3D U-Net extension: 3D U-Net is a simple extension of U-Net for 3D image segmentation, using three downsampling operations and batch normalization after each convolutional layer, but neither 3D U-Net nor U-Net uses dropout. The number of channels is doubled before both upsampling and downsampling to avoid bottlenecks.
作为一篇介绍U-Net网络及其变体的文章,该文对于U-Net的结构和应用场景进行了简要概述,并提到了U-Net在医学图像分割中的优势。然而,该文存在以下几个问题:
1. 片面报道:该文只介绍了U-Net网络及其变体,在介绍其他相关工作时只是简单提到,并未深入探讨。这样可能会给读者留下片面的印象,认为U-Net是唯一可行的解决方案。
2. 缺失考虑点:该文没有提到U-Net在处理大规模数据时可能会遇到的问题,如内存限制、计算时间等。这些问题可能会影响U-Net在实际应用中的效果。
3. 偏袒:该文对于U-Net网络持有较为偏袒的态度,并未探讨其缺点和局限性。这样可能会误导读者,使他们过分依赖于U-Net网络。
4. 宣传内容:该文中出现了一些宣传性质的内容,如“UNet's skip connection and U-shaped structure come in handy”、“The U-Net architecture achieves very good performance in different biomedical segmentation applications”。这些内容并未给出具体证据支持,容易引起读者怀疑。
5. 未探索反驳:该文没有探讨其他学者对于U-Net网络及其变体的批评和反驳意见。这样可能会使读者对于U-Net网络存在一定程度上的误解。
总之,虽然该文对于U-Net网络及其变体进行了简要介绍,但是存在以上几个问题需要进一步完善和改进。