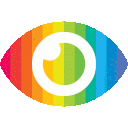
1. Depth image enhancement is crucial for many high-level 3D vision tasks, but the captured depth images from commercial depth sensors still suffer from various quality issues.
2. Traditional RGB-guided methods for depth image enhancement have limitations due to their reliance on prior assumptions/models and the difficulty in collecting real-world noisy-clean pairs for supervised learning.
3. The proposed self-supervised learning method exploits the dependency between RGB and depth modalities to significantly boost the enhancement performance on real-world noisy depth images without requiring any noisy-clean pairs. The method also utilizes an augmentation of the cross-modal dependency maximization formulation based on optimal transport theory to achieve further performance improvement.
该文章是一篇关于自监督学习在RGB引导下深度增强中的应用的研究论文。文章提出了一个新颖的自监督学习方法,利用RGB和深度之间的依赖关系来自我监督增强模型的训练。此外,文章还提出了一种基于最优传输理论的跨模态依赖性最大化公式的增强方法,以实现更好的性能。
然而,该文章存在一些潜在偏见和不足之处。首先,文章没有探讨可能存在的风险或负面影响。其次,文章没有平等地呈现双方观点,并且可能存在宣传内容。此外,文章未提供足够的证据来支持其所提出主张,并且可能存在片面报道和缺失考虑点。
总之,尽管该论文提出了一个有前途的方法来解决深度图像增强问题,但需要更多研究来验证其有效性并充分考虑潜在风险和局限性。