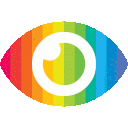
1. The integration of robots into society requires the revision of human-robot interactions to make it as seamless and natural as possible.
2. Emotion recognition is an emerging approach to modeling social behaviors for service robots, which can be used for dictating a robot's behavior and associating emotions to events or objects in specific domains.
3. A framework for emotion detection based on text analysis for social robots has been proposed, which allows social robots to detect emotions and store this information in an ontology-based repository called EMONTO (an EMotion ONTOlogy).
The article "Emotion Detection for Social Robots Based on NLP Transformers and an Emotion Ontology" presents a framework for emotion recognition in social robots based on natural language processing (NLP) transformers and an emotion ontology. The authors argue that emotion recognition is essential to improving human-robot interactions, and their proposed framework allows robots to detect emotions in real-time via different media, such as text, speeches, images, or videos. The detected emotions are stored in a semantic repository based on an extensible ontology called EMONTO, which represents emotions and can be extended with other specific domain ontologies.
The article provides a comprehensive review of recent studies related to social robots and emotion detection from speech and text. However, the authors focus mainly on the benefits of their proposed framework without discussing potential risks or limitations. For example, they do not address concerns about privacy or data security when storing emotional information in a semantic repository. Additionally, the article does not explore counterarguments or alternative approaches to emotion recognition in social robots.
The authors present a case study of tour-guide robots for museums to demonstrate the suitability of their approach. They use NLP transformers to make sentence embeddings and classify emotions in texts obtained directly from people or by converting speech into text. The results show acceptable performance compared with state-of-the-art transformer-based models applied in the same task.
Overall, the article provides valuable insights into the potential benefits of using NLP transformers and an emotion ontology for emotion recognition in social robots. However, it would benefit from addressing potential risks and limitations associated with storing emotional information in a semantic repository and exploring alternative approaches to emotion recognition.