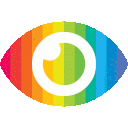
1. Intracranial aneurysms are a serious neurological condition that can lead to subarachnoid hemorrhage, with high mortality and morbidity rates.
2. Current methods for assessing the rupture risk of aneurysms rely on subjective evaluations by physicians, leading to inconsistency and potential risks associated with surgery.
3. This study explores the use of multidimensional features derived from high-resolution angiographic images and three-dimensional aneurysm modeling data to build a semiautomatic prediction model for rupture risk estimation of aneurysms.
The article titled "Intracranial Aneurysm Rupture Risk Estimation With Multidimensional Feature Fusion" provides an overview of the use of machine learning and radiomics in predicting the rupture risk of intracranial aneurysms. While the article presents valuable information on the topic, there are several points that need to be critically analyzed.
One potential bias in the article is the lack of discussion on the limitations and potential risks associated with using machine learning and radiomics for rupture risk assessment. The article focuses primarily on the benefits and potential of these technologies but does not adequately address their limitations or potential pitfalls. For example, it does not mention that machine learning models are only as good as the data they are trained on, and if there are biases or errors in the training data, it can lead to inaccurate predictions. Additionally, there is no discussion on the ethical considerations of using AI algorithms in medical decision-making.
Another point to consider is that the article mainly presents evidence supporting the use of machine learning and radiomics for rupture risk assessment but does not provide a balanced view by discussing any potential drawbacks or criticisms of these approaches. It would have been beneficial to include a section discussing any existing criticisms or concerns raised by experts in the field regarding these methods.
Furthermore, while the article mentions that doctors currently assess rupture risk based on high-resolution angiographic images and patient-specific clinical factors, it does not provide any evidence or references to support this claim. Without supporting evidence, this claim remains unsupported and raises questions about its validity.
Additionally, there is a lack of discussion on how these predictive models would be integrated into clinical practice. The article briefly mentions that a computer-aided diagnosis system could assist doctors in diagnosis and decision-making but does not elaborate on how this integration would occur or what challenges may arise from implementing such a system.
Moreover, there is no mention of potential conflicts of interest or funding sources for the research presented in the article. It is important to consider any potential biases that may arise from financial or other conflicts of interest.
In conclusion, while the article provides valuable information on the use of machine learning and radiomics for rupture risk assessment of intracranial aneurysms, there are several points that need to be critically analyzed. These include potential biases, one-sided reporting, unsupported claims, missing evidence, unexplored counterarguments, and a lack of discussion on limitations and potential risks associated with these approaches. A more balanced and comprehensive analysis would have strengthened the article's credibility and provided a more nuanced understanding of the topic.