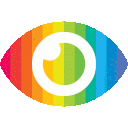
1. The manual analysis of ultrasound images of ovaries for follicle detection can be tedious and lead to erroneous results.
2. A method using K-means clustering is proposed for automatic segmentation of follicles from ultrasound images.
3. The detection of follicles in ultrasound images can aid in the diagnosis and monitoring of diseases like infertility, PCOS, and ovarian cancer.
The article titled "Follicle Segmentation Using K-Means Clustering from Ultrasound Image of Ovary" discusses a method for automatically segmenting follicles from ultrasound images using the K-means clustering technique. The authors highlight the importance of accurately detecting and analyzing follicles in the ovary for the diagnosis and monitoring of various diseases such as infertility, PCOS, and ovarian cancer.
One potential bias in this article is the lack of discussion on the limitations or challenges associated with using the K-means clustering technique for follicle segmentation. While the authors mention that manual analysis can be tedious and lead to erroneous results, they do not address whether their proposed method is free from these limitations. It would have been beneficial to include a discussion on potential issues such as noise in ultrasound images, variability in follicle appearance, or overlapping follicles that may affect the accuracy of segmentation using K-means clustering.
Additionally, the article does not provide a comprehensive review of existing techniques for follicle detection and segmentation. Although it references a few related studies, it fails to discuss other relevant methods or compare their performance with the proposed approach. This omission limits the reader's understanding of how this method compares to alternative techniques and whether it offers any advantages or improvements.
Furthermore, there is no mention of any potential risks or drawbacks associated with automated follicle segmentation using ultrasound images. It would have been valuable to discuss any possible false positives or false negatives that could arise from this method and how they might impact clinical decision-making.
The article also lacks evidence to support its claims about the effectiveness of the proposed method. While it briefly mentions that K-means clustering is used for segmentation, there is no detailed explanation or validation provided. The absence of experimental results or comparisons with ground truth annotations makes it difficult to assess the accuracy and reliability of this approach.
Moreover, there is a lack of exploration of counterarguments or alternative perspectives on automated follicle segmentation. The article presents the proposed method as a solution without acknowledging any potential criticisms or limitations that may exist.
In terms of promotional content, the article does not appear to have any overt bias towards a particular product or service. However, it is worth noting that the authors acknowledge Gama Imaging and Diagnostic Center for providing the ultrasound images and Dr. Md. Laskar Ali for manually segmenting follicle regions. This acknowledgment could potentially introduce a bias towards these entities or their associated practices.
Overall, this article provides an overview of a method for follicle segmentation using K-means clustering but lacks critical analysis, evidence, and discussion of alternative approaches. It would benefit from addressing potential limitations, providing validation results, and considering counterarguments to present a more balanced perspective on automated follicle segmentation in ultrasound images.