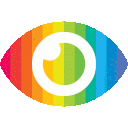
1. Music-streaming services have grown dramatically, creating the challenge of finding songs that fit users' tastes.
2. Deep learning has shifted the paradigm in music classification and tagging from feature engineering to end-to-end learning.
3. CNNs have been the most widely used learning model in music classification and tagging tasks, with recent successful attempts using raw waveforms as input.
The article "Deep Learning for Audio-Based Music Classification and Tagging: Teaching Computers to Distinguish Rock from Bach" provides a comprehensive overview of the use of deep learning in music classification and tagging. The article highlights the challenges faced by music streaming services in recommending songs to users based on their preferences and discusses how deep learning can be used to automate the process of classifying music content.
The article is well-researched and provides a detailed analysis of the different approaches to feature engineering and feature learning in music classification. It also discusses the limitations of traditional machine learning frameworks and how deep neural networks have opened up new possibilities for end-to-end learning.
However, there are some potential biases in the article that need to be considered. For example, the article focuses primarily on the benefits of deep learning for music classification and tagging, without discussing any potential risks or drawbacks. Additionally, while the article acknowledges that hand-engineered features have advantages in terms of interpretability, it does not explore whether these features may still have value in certain contexts.
Furthermore, while the article provides a detailed overview of different CNN models for music classification, it does not discuss any alternative approaches or counterarguments. This could give readers an incomplete understanding of the field.
Overall, while this article provides valuable insights into the use of deep learning for music classification and tagging, readers should approach it with a critical eye and consider other perspectives as well.