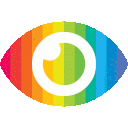
1. A systematic review and meta-analysis was conducted to assess the diagnostic accuracy of artificial intelligence (AI) algorithms in detecting cerebral aneurysms using CT, MRI, or DSA.
2. The analysis included 43 studies, with most using AI as a standalone tool. The overall sensitivity for standalone AI was 91.2%, while for reader-assistive AI it was 90.3%.
3. However, the study found that most studies had a high risk of bias and poor generalizability, limiting the interpretation of the results. Multicenter, prospective studies are needed to further evaluate the use of AI in clinical practice for detecting cerebral aneurysms.
The article titled "Detection of cerebral aneurysms using artificial intelligence: a systematic review and meta-analysis" provides a comprehensive analysis of the diagnostic accuracy of artificial intelligence (AI) algorithms in detecting cerebral aneurysms using various imaging modalities. While the study presents valuable insights into the potential of AI in improving aneurysm detection, there are several points that need to be critically analyzed.
One potential bias in the study is the inclusion of predominantly retrospective studies. Out of the 43 studies included, 41 were retrospective, which may introduce selection bias and limit the generalizability of the findings. Additionally, most studies had a high risk of bias and concerns regarding applicability, which further limits the strength of the conclusions drawn from this review.
The article acknowledges that AI tools for aneurysm detection are not ready for routine clinical practice due to their high false-positive rates and limited evidence supporting their use. However, it also suggests that AI has the potential to support clinicians in detecting cerebral aneurysms based on bivariate per-patient analysis. This claim seems contradictory to the overall findings of high bias risk and poor generalizability.
Furthermore, while the article highlights the need for large and representative datasets in developing AI tools and subsequent validation through prospective multicenter studies, it does not provide sufficient evidence or examples of such studies. The lack of concrete evidence supporting these claims raises questions about their validity.
The article also fails to explore potential counterarguments or limitations associated with AI-based aneurysm detection. For example, it does not discuss potential ethical concerns related to relying solely on AI algorithms for diagnosis or address issues related to algorithm transparency and interpretability.
Additionally, there is a lack of discussion on possible risks or limitations associated with implementing AI tools in clinical practice. The article briefly mentions increased workload and cost due to high false-positive rates but does not delve into other potential risks such as over-reliance on AI, potential biases in the training data, or the need for continuous monitoring and updating of AI algorithms.
The article also does not present both sides of the argument equally. While it acknowledges the limitations and challenges associated with AI-based aneurysm detection, it emphasizes the potential benefits without providing sufficient evidence to support these claims.
In conclusion, while the article provides valuable insights into the use of AI in detecting cerebral aneurysms, there are several biases and limitations that need to be critically analyzed. The high risk of bias in the included studies, limited evidence supporting AI use, and lack of exploration of counterarguments and potential risks raise questions about the validity and generalizability of the findings. Further research is needed to address these limitations and provide a more comprehensive understanding of the role of AI in aneurysm detection.