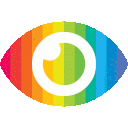
1. Signal processing on graphs is an emerging field that merges algebraic and spectral graph theoretic concepts with computational harmonic analysis to process high-dimensional data on the vertices of weighted graphs.
2. Graph spectral domains are defined as analogues to the classical frequency domain, and it is important to incorporate the irregular structures of graph data domains when processing signals on graphs.
3. Methods have been developed to generalize fundamental operations such as filtering, translation, modulation, dilation, and downsampling to the graph setting, and localized multiscale transforms have been proposed to efficiently extract information from high-dimensional data on graphs.
The article titled "The Emerging Field of Signal Processing on Graphs: Extending High-Dimensional Data Analysis to Networks and Other Irregular Domains" provides a comprehensive overview of the field of signal processing on graphs. The authors outline the main challenges in this area, discuss different ways to define graph spectral domains, and highlight the importance of incorporating the irregular structures of graph data domains when processing signals on graphs.
One potential bias in this article is that it focuses primarily on the benefits and potential applications of signal processing on graphs, without discussing any potential risks or drawbacks. While it is true that this field has many exciting possibilities, it is important to acknowledge that there may also be limitations or negative consequences associated with these methods.
Another issue with this article is that it does not provide much evidence for some of its claims. For example, the authors state that "localized, multiscale transforms have been proposed to efficiently extract information from high-dimensional data on graphs," but they do not provide any specific examples or studies to support this claim.
Additionally, while the authors do discuss some possible extensions and open issues in this field, they do not explore any counterarguments or alternative perspectives. This could lead readers to believe that there is only one way to approach signal processing on graphs, when in reality there may be multiple valid approaches depending on the specific application or context.
Overall, while this article provides a useful introduction to signal processing on graphs, it would benefit from more balanced reporting and a deeper exploration of potential limitations and alternative perspectives.